Compound V3 Economic Audit Announcement
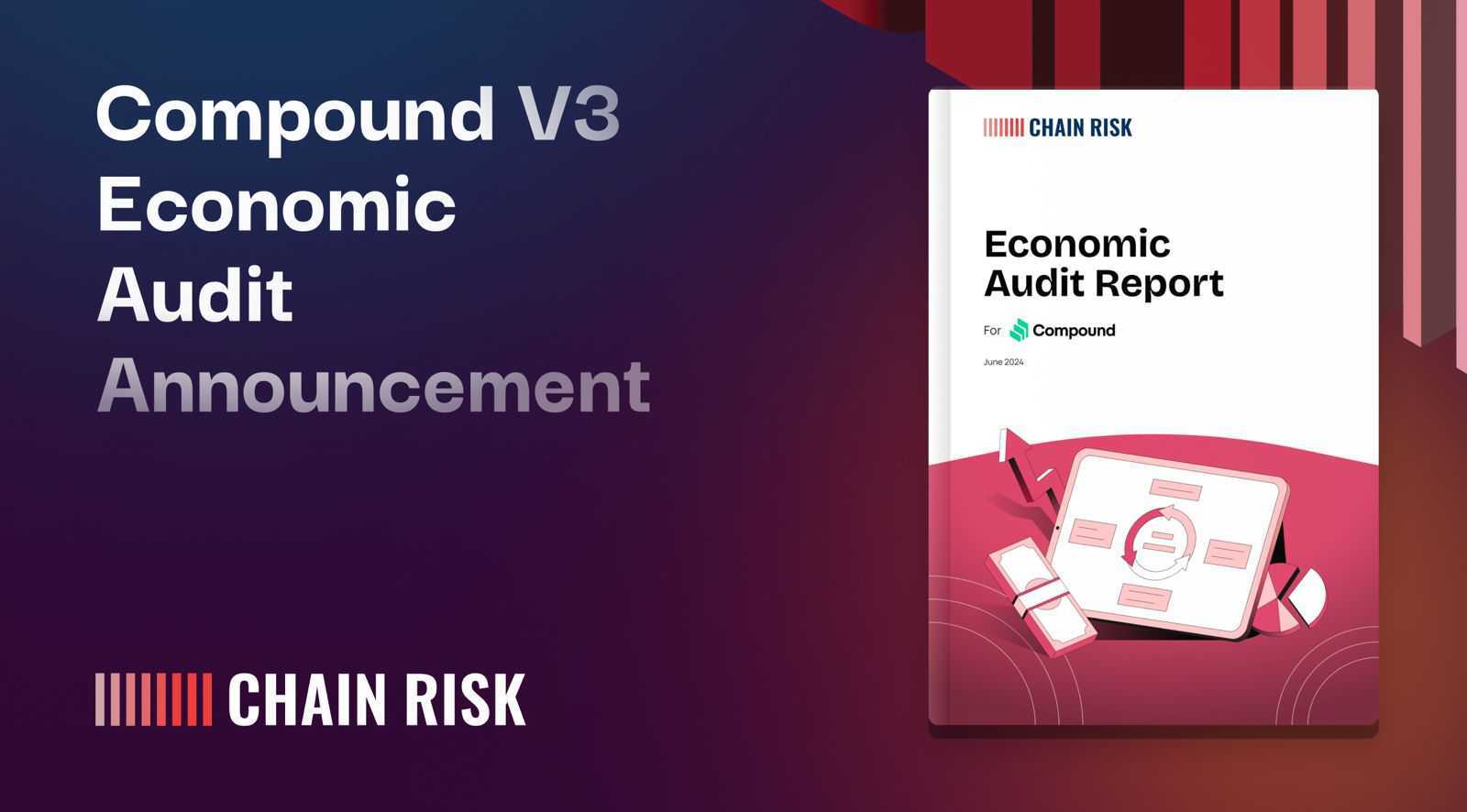
This economic audit focuses on leveraging Chainrisk’s advanced simulation engine to optimize the risk parameters for Compound V3. This milestone plays a pivotal role in ensuring that the protocol operates efficiently while mitigating risks. The emphasis is on enhancing the overall stability of the protocol by stress testing it under different market conditions, particularly on Arbitrum One Chain within the USDC market.
The primary goal is to establish a robust market risk calculation framework for Compound V3. Chainrisk has applied its simulation platform to determine optimal risk parameters that strike a balance between capital efficiency and protocol risk. By stress testing the protocol, Chainrisk aims to:
- Assess the resilience of the Compound V3 protocol under extreme market scenarios.
- Calculate key metrics, such as Value at Risk (VaR) and Liquidations at Risk (LaR), specifically for the USDC market on Arbitrum.
- Recommend risk parameters based on the simulation results to optimize the protocol’s performance and stability.
- Mitigate potential systemic risks by tailoring these recommendations to improve the robustness of the Compound protocol’s risk exposure.
Scope of the Economic Audit
The economic audit performed by Chainrisk in this milestone focuses specifically on the USDC market on the Arbitrum One Chain. This market has become increasingly significant as a hub for borrowing and lending in decentralized finance (DeFi) protocols, including Compound. The audit is aimed at analyzing the stability and risk parameters of the assets within this market, which are critical to maintaining the market’s liquidity and ensuring its resilience against volatility.
The scope of the audit extends to evaluating the collateral assets that are key components of the USDC market on Arbitrum. These include:
- Wrapped Ether (WETH)
- Wrapped Bitcoin (WBTC)
- GMX
- Arbitrum (ARB)
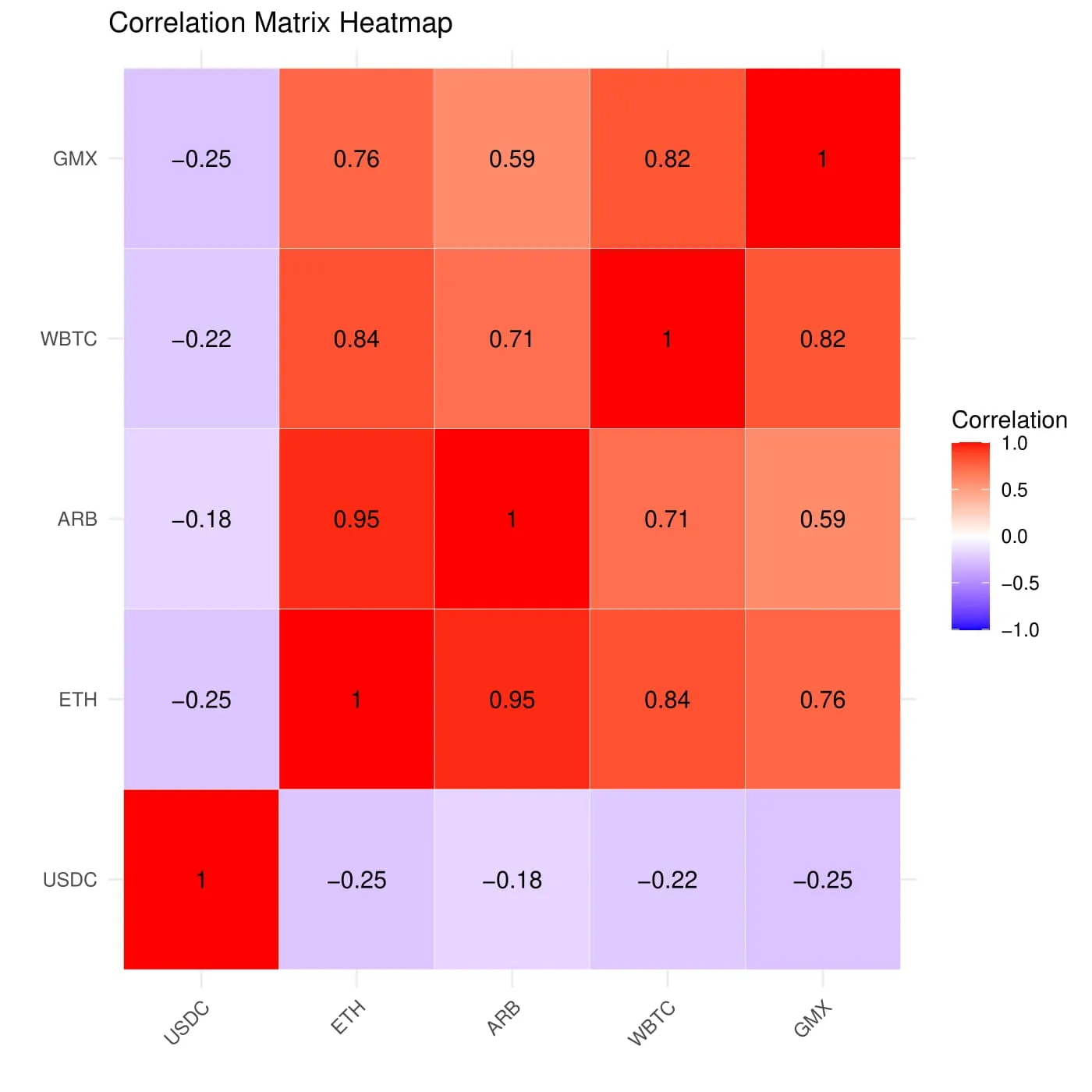
Each of these assets plays a vital role as collateral in the borrowing and lending processes within the protocol. The objective of the audit is to analyze the risk exposure of each of these collateral types and how their behavior in various market conditions could affect the stability of the Compound V3 protocol. Chainrisk specifically focuses on stress testing these assets to provide a comprehensive understanding of the risk levels they introduce to the USDC market.
In this phase, the analysis looks at how these assets perform during adverse conditions, such as market crashes or high volatility periods, and how their risk parameters could be optimized to protect the Compound protocol from systemic risks.
Chainrisk Simulation Engine
The Chainrisk Simulation Engine is a core component of the analysis performed in this milestone. It is a modular testing environment specifically configured to align with the existing state of Compound V3, including various actors like lenders, borrowers, liquidators, contracts, and oracles. This engine is designed to conduct high-fidelity simulations that mirror real-world market scenarios, allowing Chainrisk to identify potential risks and optimize parameters accordingly.
Components of the Simulation Engine:
A. RiskEVM (Rust-based Simulation): RiskEVM is a high-performance, Rust-based simulation engine that is optimized for handling heavy computational tasks. This component is responsible for running complex simulations that require significant processing power, making it ideal for analyzing intricate financial systems like Compound. It focuses on modeling the interactions within the protocol, including borrowing, lending, and liquidation events, under various simulated market conditions. By performing these computations, RiskEVM helps in assessing the protocol’s behavior and stability, especially during periods of market stress.
B. On-Chain Simulation: This component of the engine is responsible for conducting backtests on forked networks, ensuring that the simulations are as accurate and factual as possible. This part of the engine is designed to replicate real-world scenarios by using data from actual on-chain activity. By running simulations on these forked versions of the network, the engine can evaluate how the Compound protocol would react under different conditions, providing insights into its resilience and potential weaknesses. This level of testing ensures that the risk parameter recommendations are well-grounded in realistic scenarios.

The combination of these two components allows the Chainrisk Simulation Engine to deliver a robust analysis of the Compound V3 protocol’s risk exposure. The engine’s ability to mirror real-world conditions helps ensure that the analysis is not only theoretical but also practically applicable, enhancing the protocol’s stability and capital efficiency on the Arbitrum One Chain.
Methodology for Risk Parameter Optimization
The methodology employed by Chainrisk for optimizing risk parameters in the Compound V3 protocol focuses on a careful balance between capital efficiency and protocol risk. The approach involves a detailed analysis of market behavior, stress testing, and simulation-based evaluations to derive the most effective risk parameters for the protocol.
The optimization framework is designed to ensure that the protocol remains capital efficient without compromising on risk management. Capital efficiency refers to the ability of the protocol to maximize returns for users (e.g., lenders and borrowers) while minimizing the amount of capital that is locked as collateral. However, prioritizing efficiency should not lead to excessive risk-taking that could destabilize the protocol.
Chainrisk's methodology seeks to find a balance where the protocol can function efficiently, allowing for high capital utilization, while also setting risk parameters that prevent potential vulnerabilities, particularly during market downturns.
The risk calculation framework developed by Chainrisk uses a combination of agent-based simulations and statistical models to assess the market risk associated with the Compound V3 protocol. The framework incorporates historical data, real-time on-chain activity, and potential future market conditions to develop comprehensive risk profiles for different assets and scenarios.
Key elements of this framework include:
- Value at Risk (VaR): A statistical measure that estimates the maximum potential loss the protocol could face under adverse market conditions over a specified time frame.
- Liquidations at Risk (LaR): Similar to VaR, LaR focuses on the risk of liquidations within the protocol, helping to understand the likelihood of collateral being sold off under stress.
To evaluate the robustness of the protocol, Chainrisk employs stress testing to simulate how the protocol would perform under extreme or adverse market conditions. This involves running multiple simulations that account for various potential scenarios, including sharp declines in asset prices, sudden spikes in borrowing activity, or changes in the liquidity of collateral assets.
The goal of stress testing is to identify weaknesses in the protocol’s current setup and provide insights into how the risk parameters could be adjusted to improve resilience. This could include recommendations on collateral requirements, borrowing limits, and liquidation thresholds, among other factors.
By using this methodology, Chainrisk aims to provide tailored recommendations that ensure the Compound V3 protocol remains stable, secure, and efficient, even under challenging market conditions. The optimization framework allows the protocol to adapt to changing market dynamics while maintaining a high level of performance and security.
Agent-Based Monte Carlo Simulations
Agent-based Monte Carlo simulations are a key component of Chainrisk’s risk assessment methodology for the Compound V3 protocol. These simulations allow for detailed modeling of the interactions between various agents (e.g., borrowers, lenders, and liquidators) within the protocol, helping to estimate potential losses and understand risk dynamics under different market scenarios.
The simulation process models the behavior of two key types of agents:
- Borrowers: These agents are modeled based on historical on-chain data. The simulation tracks how borrowers adjust their positions based on changing market conditions, such as fluctuations in collateral value or interest rates. The behavior of borrowers is critical in understanding the protocol's risk exposure, especially during periods of high volatility.
- Liquidators: Liquidators are responsible for executing liquidations when borrowers fail to maintain sufficient collateral. The simulations include a profit function for liquidators that takes into account various factors such as slippage, trading fees, and liquidation penalties. This helps in understanding the conditions under which liquidations would occur and how they would impact the protocol’s stability.
One of the key challenges in risk modeling is that a single simulation may not capture all possible outcomes, especially in a complex system like Compound. Therefore, Chainrisk uses a multi-simulation approach:
- Multiple Iterations: Each simulation represents a different set of potential market conditions, and by running thousands of these simulations, Chainrisk can account for a broad range of scenarios.
- Unbiased and Independent Results: By ensuring that each simulation is statistically unbiased and independent, the results provide a more accurate estimate of the expected value of potential losses. This allows Chainrisk to generate a distribution of outcomes, which is crucial for determining risk metrics like VaR (Value at Risk) and LaR (Liquidations at Risk).
The multi-simulation approach provides a comprehensive understanding of how the protocol might perform under various future conditions, including rare but extreme events. This helps in identifying potential weaknesses and crafting risk management strategies that are robust across a range of possible scenarios.
Slippage and Trading Analysis
Slippage plays a significant role in determining the behavior of trades, particularly during liquidation events. Slippage refers to the difference between the expected price of a trade and the actual price at which the trade is executed. For borrowers and liquidators, understanding slippage is critical as it affects the overall costs and profitability of transactions.
Chainrisk developed a slippage model to analyze how slippage impacts trading activities within the protocol. The model aimed to:
- Identify Patterns in Slippage: By analyzing historical trading data, the model detected patterns in slippage rates across different assets. Understanding these patterns helped in predicting how slippage might change under varying market conditions.
- Optimize Trading Execution: The slippage model provided insights into how trades could be executed more efficiently, minimizing costs for participants and reducing the risk of negative impacts on the protocol’s liquidity.
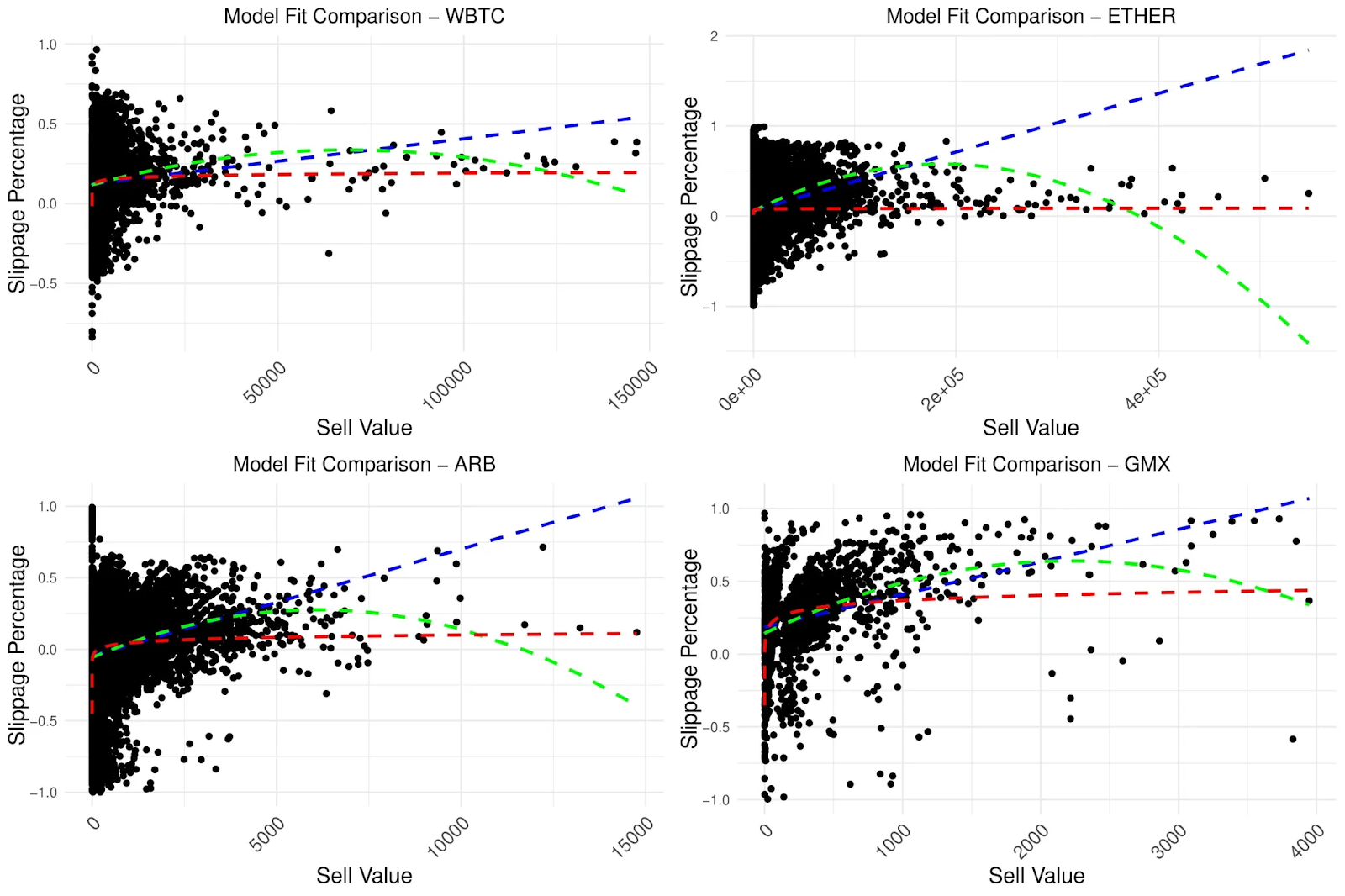
The model fit was assessed using efficiency statistics, such as Mean Squared Error (MSE), to ensure that it accurately reflected real-world trading behavior. This allowed Chainrisk to make more informed recommendations on risk parameters, especially around conditions that could lead to high slippage during times of market stress.
By analyzing slippage data and its correlation with trading activity, Chainrisk was able to provide a deeper understanding of how market conditions might affect the protocol’s risk exposure. This helped in developing models that could predict slippage levels based on different scenarios, enabling the creation of more robust risk parameters for the Compound V3 protocol.
Estimating Value at Risk (VaR)
Value at Risk (VaR) is a critical metric used to estimate the maximum potential loss a protocol like Compound V3 might incur under adverse market conditions within a specified confidence interval. Chainrisk utilized a simulation-based approach to calculate VaR, ensuring that the assessment was both accurate and reliable by simulating a broad range of possible scenarios.
The process of estimating VaR through simulations involved multiple steps to ensure robustness and accuracy:
- Initial Simulation: Chainrisk conducted an initial set of 5,000 simulations using estimated parameters. Each simulation represented a different set of potential market conditions, including changes in asset prices, borrowing behavior, and liquidity levels.
- Convergence Check: To confirm the reliability of the initial estimate, Chainrisk ran an additional 5,000 simulations with the same parameters. These new results were combined with the initial set, creating a total of 10,000 simulations.
- Final Calculation: Once convergence was achieved, Chainrisk conducted a final set of 5,000 simulations, bringing the total number of simulations to 15,000. This comprehensive dataset allowed for a more robust calculation of VaR.
The convergence check was a crucial part of the process, ensuring that the VaR estimates were not sensitive to fluctuations in the data. By running multiple rounds of simulations and confirming that the results remained consistent, Chainrisk ensured that the risk assessment was reliable and could be used for making informed decisions about setting risk parameters for the protocol.
The VaR calculation provided a comprehensive view of the potential risk exposure of the Compound V3 protocol. The simulation-based VaR approach allowed Chainrisk to:
- Identify risk exposure across different assets and market conditions, enabling a more targeted approach to managing risk.
- Optimize collateral and liquidation parameters, ensuring that the protocol maintained adequate security even during periods of high volatility.
- Prepare for adverse scenarios by estimating the maximum potential loss and developing contingency plans to handle such events.
The use of multiple simulations ensured that the estimates were accurate, stable, and reflective of potential market conditions, allowing for more informed and effective risk management strategies.
Results and Observations
The results of Chainrisk’s analysis provided key insights into the risk exposure and resilience of the Compound V3 protocol under various market scenarios. The simulations conducted throughout the study yielded detailed data on Value at Risk (VaR) and Liquidations at Risk (LaR), allowing Chainrisk to make precise recommendations for optimizing risk parameters.
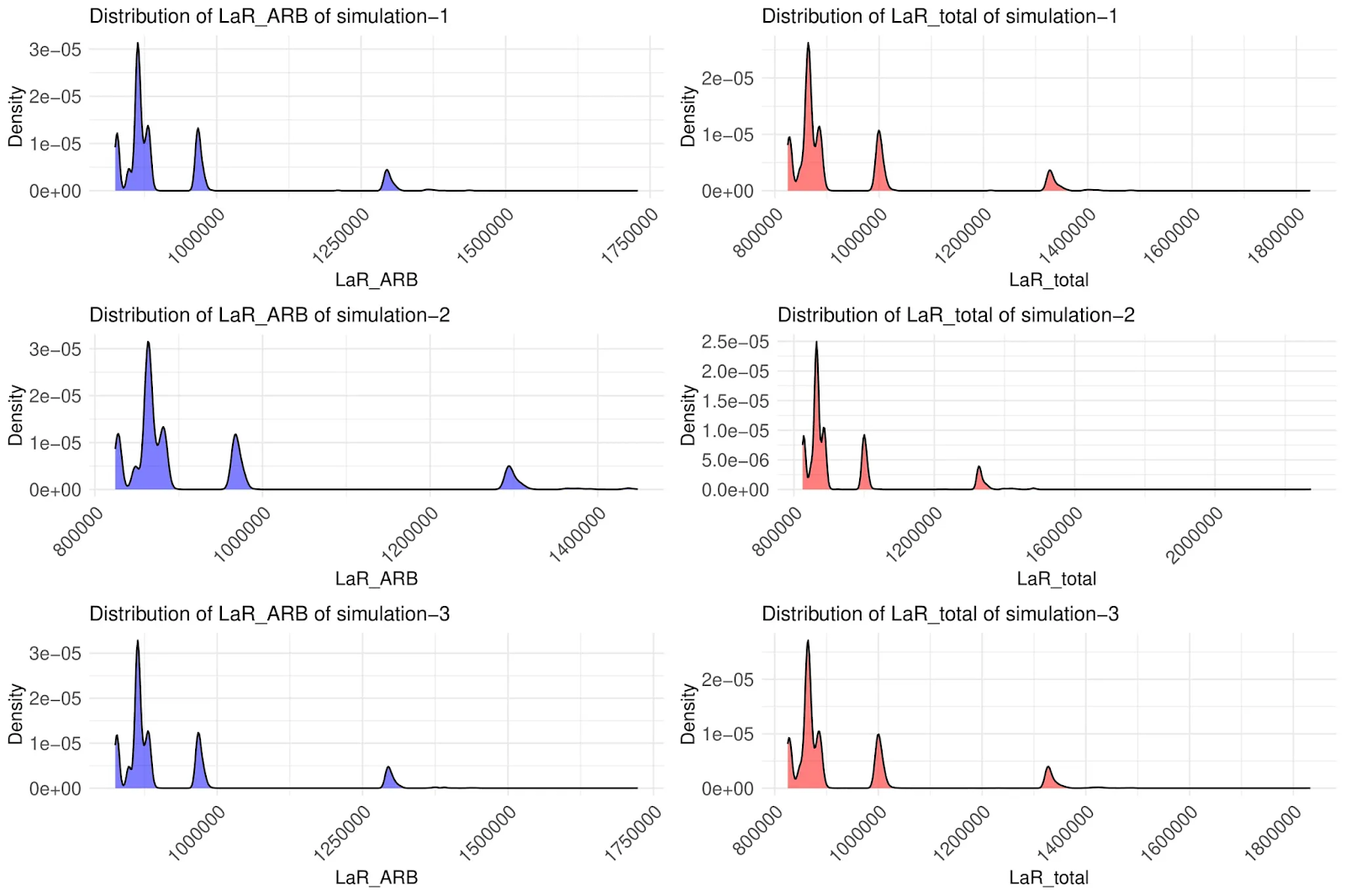
The analysis calculated both VaR and LaR for different collateral assets, including WETH, WBTC, GMX, and ARB, within the Arbitrum USDC market. The simulations revealed:
- Consistent Risk Characteristics: Across the simulations, it was observed that the risk characteristics were relatively consistent, as indicated by the nearly identical LaR distribution curves. This suggested that the protocol had similar risk exposure across different asset classes, reflecting uniform behavior under stressed conditions.
- Scenario Independence: Each simulation generated scenarios independently, which helped ensure that the risk assessments were unbiased and reflective of a wide range of potential market conditions.
In conclusion, the results and observations from the simulations provided a comprehensive understanding of how the Compound V3 protocol would behave under different market scenarios.


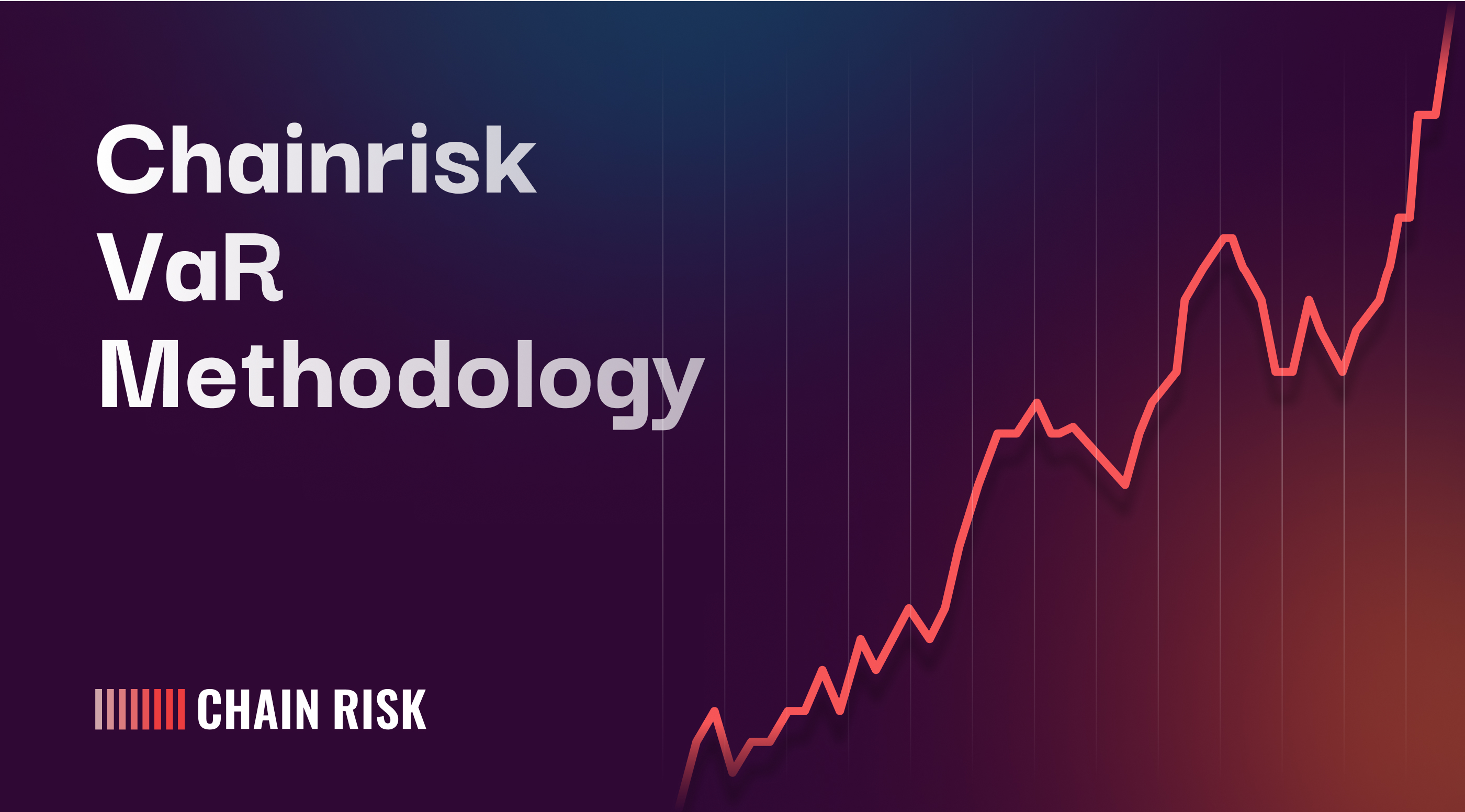
Chainrisk VaR Methodology
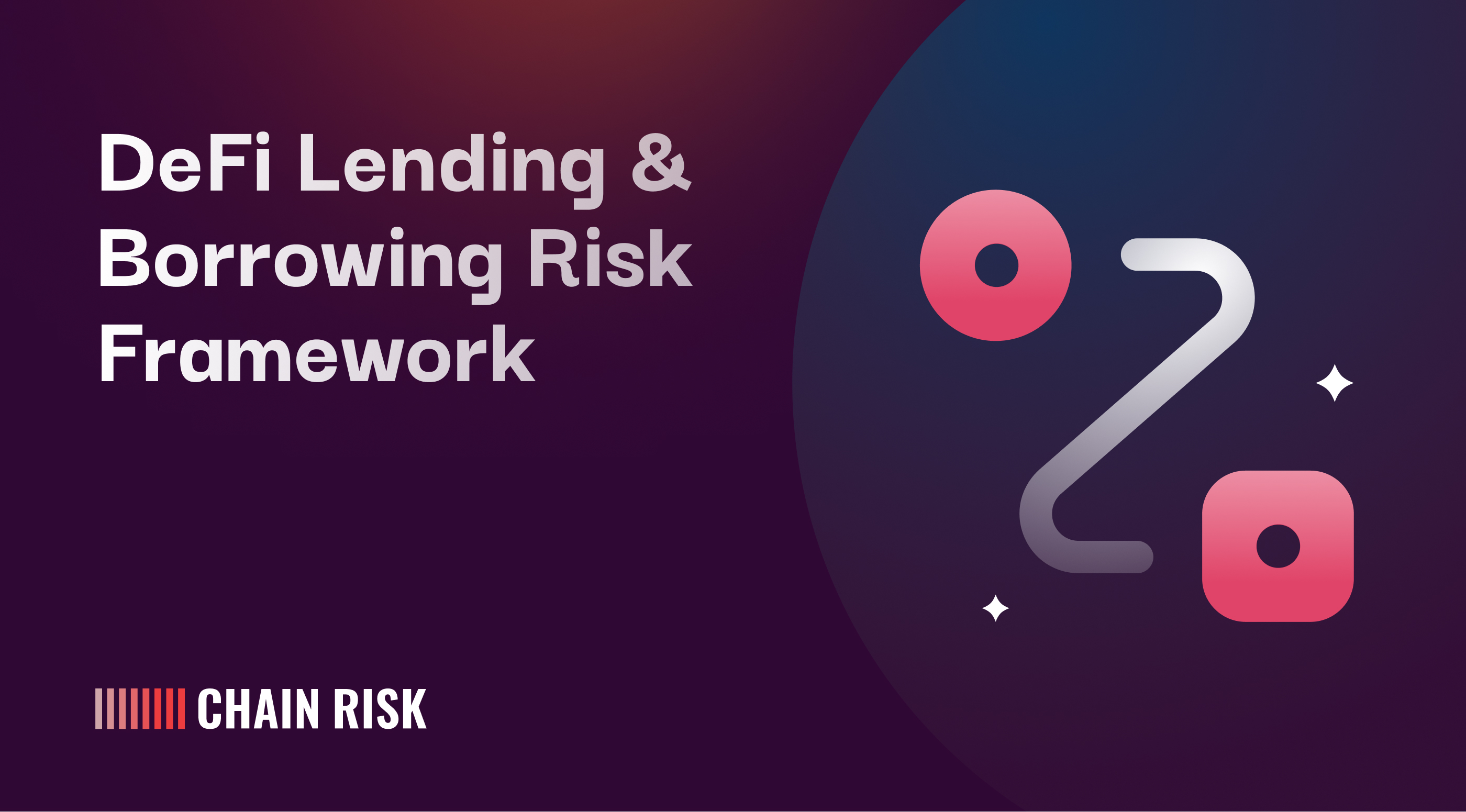
DeFi Lending & Borrowing Risk Framework
.png)